How Data Wrangling is Shaping Future of Business Analytics
How Companies Can Leverage Advanced Analytics to Combat Fraud
2025 Outlook: Data Science Careers Amid AI and Global Workforce Shifts
Leveraging Analytics And Data For Smarter Transportation Decisions

How Lightweight Frameworks Like Kafka Streams Are Simplifying Data Processing
Avoiding Common Pitfalls in Data-Driven Industries
How Cybersecurity and AI Work Together?
Role of AI in Business Intelligence— PoV
How will Generative AI transform Business Intelligence? Explore its scope in automating insights, enhancing data quality, and democratizing data access across organizations.
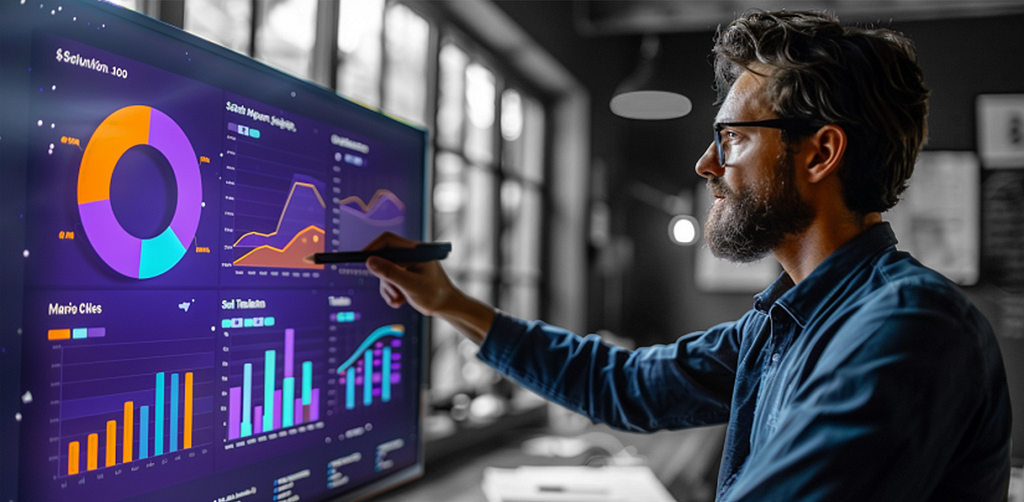
Why this blog?
Are you eager to harness the full potential of AI in your data workflows? Deep dive into the transformative power of Generative AI in Business Intelligence, empowering you to automate insights, elevate data quality, and democratize data access. Whether you’re a data scientist, analyst, or business leader, this blog offers invaluable insights to propel your organization forward in the data-driven world.
How will Generative AI transform the Business Intelligence (BI) world?
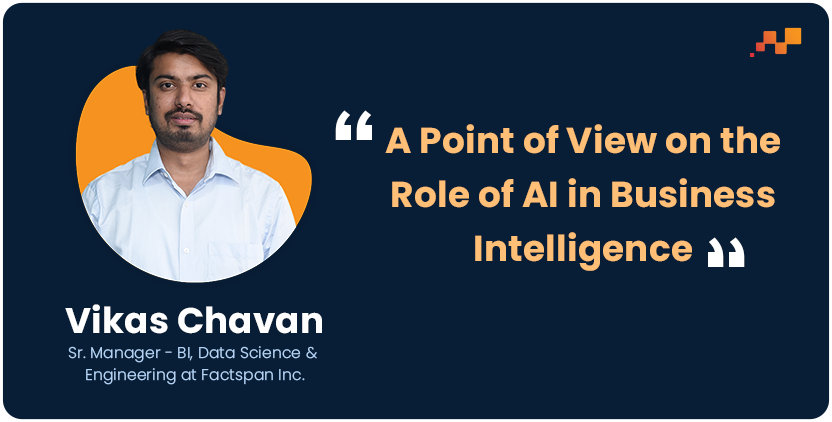
I feel, Gen AI will transform the Business Intelligence world by significantly impacting and improving the following areas:
- Text-to-SQL Automation: Generative AI converts natural language queries into SQL, making data insights accessible to everyone in the organization, not just those with technical expertise. This will speed up the decision-making process and improve the productivity of the knowledge workers.
- Automated Insights Generation & Generating visual insights: With continuous data analysis, Generative AI can automatically uncover trends, anomalies, and patterns in real time. This proactive insight generation helps businesses stay ahead of issues and seize opportunities swiftly.
- Data Synthesis and Augmentation: AI enhances data quality by generating synthetic data to fill gaps and combining multiple data sources. This creates a more comprehensive and robust dataset, leading to better insights and predictions.
- Automated data modeling and schema design — LLMs can help streamline this process, there are challenges in implementing this on a scale, though but with maturity and time, this will be improved upon.
- Data preparation and management — LLMs can play a role in the space of MDM, they can automate data cataloging making it faster and more efficient. It can continuously monitor or improve data quality by validating the anomalies.
Generative AI is set to transform Business Intelligence (BI), making it more intuitive, efficient, and powerful. This transformation, driven by Generative BI, will fundamentally change how businesses interact with and act on their data. By leveraging AI to automate tasks, uncover hidden insights, and democratize data access across the organization, Generative BI will empower all users to make more informed decisions.
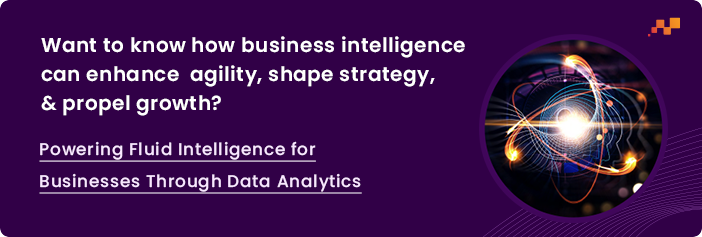
What are the primary challenges organizations face when implementing Generative BI, and how can they overcome these obstacles?
- Data Security: Ensuring data security is paramount, especially with sensitive information. Adopting privacy-preserving techniques and robust data governance frameworks can address this challenge.
- Integration Complexity: Using modular and scalable architectures facilitates the seamless integration of generative models into existing systems, reducing complexity.
- Managing User Expectations: Continuous education and setting realistic goals are crucial. Regular training sessions and workshops can familiarize users with the capabilities and limitations of Generative BI.
How can Generative BI improve operational efficiency and drive self-serving analytics and data literacy gaps for business users?
Generative BI enables business users to generate reports and dashboards without needing to write SQL queries or understand complex BI tools. By using natural language processing, Generative BI simplifies data interaction, allowing users to quickly obtain insights and make data-driven decisions independently. It can automate numerous repetitive and time-consuming tasks, significantly improving operational efficiency and driving cost savings.
For example, by automating the generation of reports and initial drafts, organizations can save substantial amounts of time and reduce personnel costs. Additionally, enhanced data analysis capabilities allow businesses to optimize their operations by identifying inefficiencies and areas for improvement, leading to further cost savings and productivity gains. We have been working on building the Insights co-pilot and have received good response from our stakeholders, it helps in generating the automated insights and visual data using NLQ.
How can organizations effectively balance the need for experimentation with Generative BI and the imperative to deliver measurable business value?
Balancing experimentation with the need to deliver measurable business value requires a strategic approach. Organizations should adopt an iterative development process, starting with small-scale pilot projects to test and refine Generative BI applications. Clear objectives and KPIs should be defined to measure the success of these experiments.
In my experience, involving cross-functional teams from the outset ensured that the projects were aligned with business goals and had practical applications. Regularly reviewing and adjusting the projects based on feedback and results helped maintain focus on delivering tangible value while we delivered these applications and kept innovating with the new advancements in this space.
How can a semantic layer improve self-service analytics when combined with Generative AI, and what challenges might organizations face in integrating semantic layers with LLMs. Do you think it will accelerate the implementation of Generative BI?
The semantic layer acts as an intermediary that unifies data across various sources, ensuring consistency in business terms and metrics. This consistency allows Generative BI tools to process and generate insights more accurately and contextually. For example, by interpreting standardized definitions, the semantic layer helps avoid discrepancies and enhances the relevance of AI-generated insights, making them more actionable for business users.
For a practical example of how Generative AI can enhance business analytics, check out our case study on Gen AI-infused business analytics for logistics queries management
Sourced from Factspan
Role of AI in Business Intelligence— PoV was originally published in Becoming Human: Artificial Intelligence Magazine on Medium, where people are continuing the conversation by highlighting and responding to this story.