Reliable AI Model Tuning : Leveraging HNSW Vector with Firebase Genkit
Instant AI Model Tuning: Leveraging HNSW Vector with Firebase Genkit for Retrieval-Augmented Generation
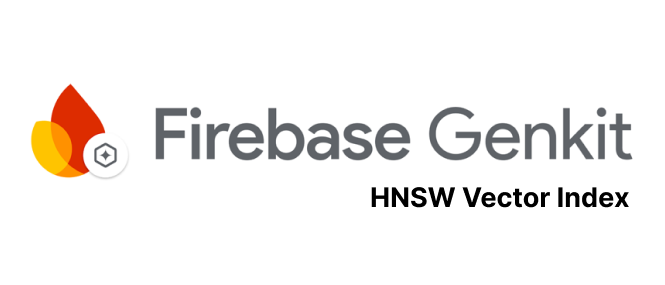
The rapid advancements in Generative AI have transformed how we interact with technology, enabling more intelligent and context-aware systems. A critical component in achieving this is Retrieval-Augmented Generation (RAG), which allows AI models to pull in specific contexts or knowledge without the need to build or retrain models from scratch.
One of the most efficient technologies facilitating this is the Hierarchical Navigable Small World (HNSW) graph-based vector index. This article will guide you through the setup and usage of the Genkit HNSW Vector index plugin to enhance your AI applications, ensuring they are capable of providing highly accurate and context-rich responses.
Understanding Generative AI
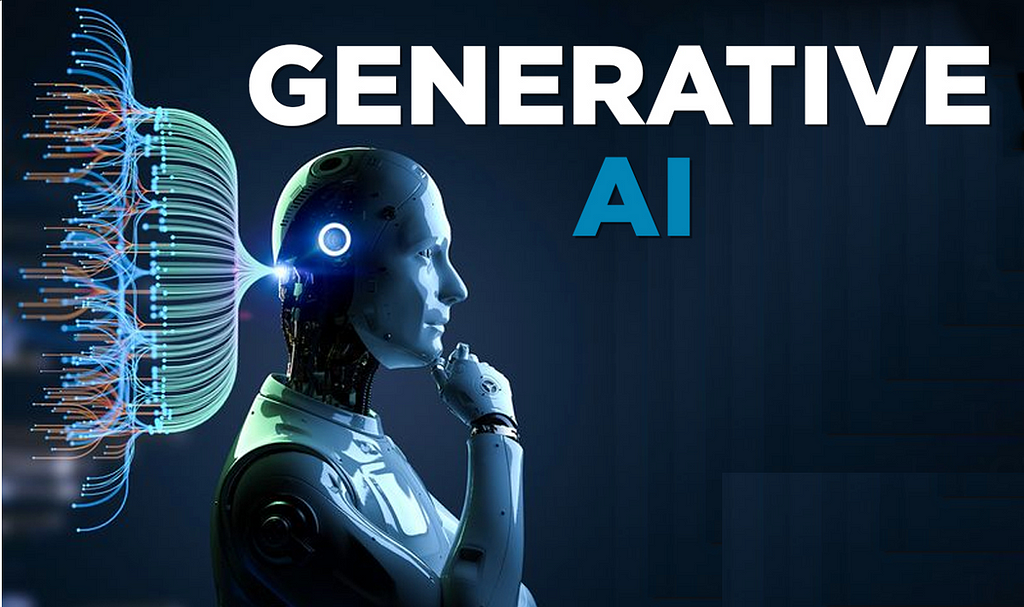
For those who still do not understand what generative AI, feel free to read about it here!
Fine-tuning in Generative AI
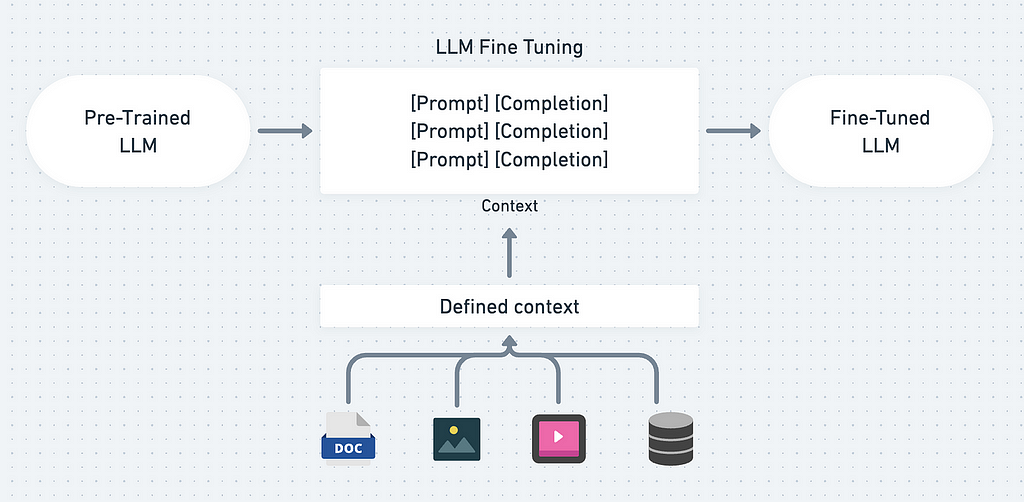
Fine-tuning is a great method to improve your AI Model! with fine-tuning, you can add more knowledge and context for the AI Model.
There are various ways to implement fine-tuning, so it is important to know how we can leverage the AI Model maximally to fit our application requirements.
If you want to read more about them and its differences, you can read more here!
Now, that we know about Generative AI and Fine-Tuning, we will learn how we can implement Retrieval-Augmented Generation (RAG) using HNSW Index.
Implementing Retrieval-Augmented Generation (RAG)
Generative AI’s capabilities can be significantly enhanced when integrated with an HNSW vector index to implement the RAG mechanism. This combination allows the AI to retrieve and utilize specific contextual information efficiently, leading to more accurate and contextually relevant outputs.
Example Use Case
Consider a restaurant application or website where specific information about your restaurants, including addresses, menu lists, and prices, is integrated into the AI’s knowledge base. When a customer inquires about the price list of your restaurant in Surabaya City, the AI can provide precise answers based on the enriched knowledge.
Example Conversation with AI Model :
You: What are the new additions to the menu this week?
AI: This week, we have added the following items to our menu:
- Nasi Goreng Kampung - Rp. 18.000
- Sate Ayam Madura - Rp. 20.000
- Es Cendol - Rp. 10.000
With RAG we can achieve a very detailed and specific response from the AI Model.
Now, to implement this, we will be using :
- HNSW Vector
We will convert our defined data into a Vector index, where it can be understood by the AI Model so that the AI Model can have a better response. - Firebase Genkit (Our special guest! :D)
We will use this to demonstrate this Retrieval-Augmented Generation (RAG) using HNSW Vector index and Gemini AI Model.
Implementing HNSW Vector index
What is HNSW?
HNSW stands for Hierarchical Navigable Small World, a graph-based algorithm that excels in vector similarity search. It is renowned for its high performance, combining fast search speeds with exceptional recall accuracy. This makes HNSW an ideal choice for applications requiring efficient and accurate retrieval of information based on vector embeddings.
Why Choose HNSW?
- Simple Setup: HNSW offers a straightforward setup process, making it accessible even for those with limited technical expertise.
- Self-Managed Indexes: Users have the flexibility to handle and manage the vector indexes on their servers.
- File-Based Management: HNSW allows the management of vector indexes as files, providing ease of use and portability, whether stored as blob or stored in a database.
- Compact and Efficient: Despite its small size, HNSW delivers fast performance, making it suitable for various applications.
Learn more about HNSW.
Implementing Firebase Genkit

What is Firebase Genkit?
Firebase Genkit is a powerful suite of tools and services designed to enhance the development, deployment, and management of AI-powered applications. Leveraging Firebase’s robust backend infrastructure.
Genkit simplifies the integration of AI capabilities into your applications, providing seamless access to machine learning models, data storage, authentication, and more.
Key Features of Firebase Genkit
- Seamless Integration: Firebase Genkit offers a straightforward integration process, enabling developers to quickly add AI functionalities to their apps without extensive reconfiguration.
- Scalable Infrastructure: Built on Firebase’s highly scalable cloud infrastructure, Genkit ensures that your AI applications can handle increased loads and user demands efficiently.
- Comprehensive Suite: Genkit includes tools for data management, real-time databases, cloud storage, authentication, and more, providing a comprehensive solution for AI app development.
Enhancing Generative AI with Firebase Genkit
By integrating Firebase Genkit with your Generative AI applications, you can significantly enhance the functionality and user experience. Here’s how Firebase Genkit contributes to the effectiveness of AI applications:
- Real-Time Data Handling: Firebase Genkit’s real-time database allows for the immediate update and retrieval of data, ensuring that your AI models always have access to the latest information. This is particularly useful for applications that require dynamic content generation based on current data, such as chatbots and recommendation systems.
- Scalable AI Deployments: Leveraging Firebase’s cloud infrastructure, Genkit enables scalable deployments of AI models. This means that as your application grows and user demand increases, the infrastructure can automatically scale to meet these needs without compromising performance.
- Simplified Data Management: With Firebase’s integrated data storage and management tools, developers can easily handle the data required for training and operating AI models. This includes capabilities for storing large datasets, real-time updates, and secure data handling.
To start using Firebase Genkit in your AI applications, follow these steps:
- Set Up Firebase: Create a Firebase project and set up your real-time database, storage, and authentication services.
- Install Genkit: Integrate Genkit into your project by following the installation instructions provided in the Genkit documentation.
- Configure Plugins: Add and configure the necessary Genkit plugins for data management, AI model integration, and user authentication.
Learn more about Firebase Genkit
Now let’s practice to learn more how we can build such an AI Solution!
Setting Up the Genkit HNSW Plugin
Prerequisites
Before installing the plugin, ensure you have the following installed:
- Node.js (version 12 or higher)
- npm (comes with Node.js)
- TypeScript (install globally via npm: npm install -g typescript)
- Genkit (install globally via npm: npm install -g genkit)
First thing first, initiate the Genkit project with
genkit init
follow the instructions here.
Once you have the Genkit project installed, make sure the project is well prepared. You can try first by
genkit start
If it runs well and open the Genkit UI in a browser, then you are good to go!
Installing the HNSW plugin
To install the Genkit HNSW plugin, run the following command:
npm install genkitx-hnsw

We will be using 2 Genkit Plugins here.
- HNSW Indexer plugin
- HNSW Retriever plugin
1. HNSW Indexer Plugin
The HNSW Indexer plugin helps create a vector index from your data, which can be used as a knowledge reference for the HNSW Retriever.
Data Preparation
Prepare your data or documents, for instance, restaurant data, in a dedicated folder.
Registering the HNSW Indexer Plugin
Import the plugin into your Genkit project:
find genkit.config.ts file in your project, usually /root/src/genkit.config.ts.
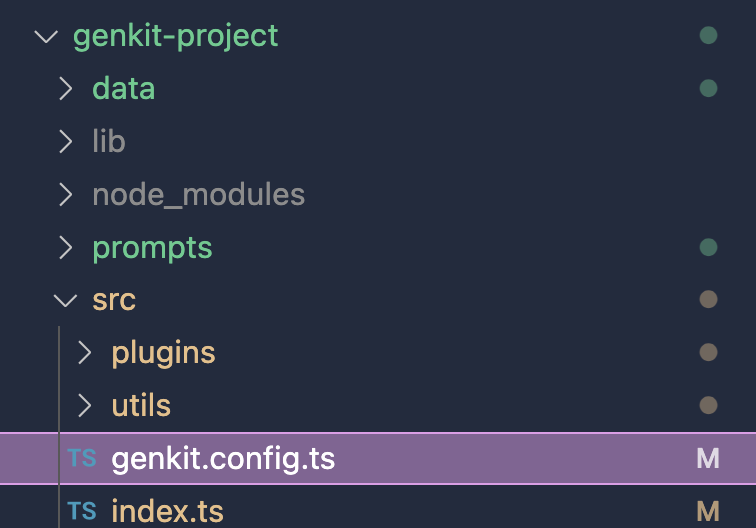
Then import the plugin into the file.
import { hnswIndexer } from "genkitx-hnsw";
//
export default configureGenkit({
plugins: [
hnswIndexer({ apiKey: "GOOGLE_API_KEY" })
]
});
Running the Indexer
- Open the Genkit UI and select the registered HNSW Indexer plugin.
- Execute the flow with the required parameters:
- dataPath: Path to your data and documents.
- indexOutputPath: Desired output path for the generated vector store index.
Vector Store Index Result
The HNSW vector store will be saved in the specified output path, ready for use with the HNSW Retriever plugin.
2. HNSW Retriever Plugin
The HNSW Retriever plugin processes prompt with the Gemini LLM Model, enriched with additional specific information from the HNSW Vector index.
Registering the HNSW Retriever Plugin
Import the necessary plugins into your Genkit project:
import { googleAI } from "@genkit-ai/googleai";
import { hnswRetriever } from "genkitx-hnsw";
export default configureGenkit({
plugins: [
googleAI(),
hnswRetriever({ apiKey: "GOOGLE_API_KEY" })
]
});
Running the Retriever
- Open the Genkit UI and select the HNSW Retriever plugin.
- Execute the flow with the required parameters:
- prompt: Your input query is for the AI.
- indexPath: Path to the vector index file generated by the HNSW Indexer plugin.
Example Prompt
To ask about the price list of a restaurant in Surabaya City:
prompt: "What is the price list of my restaurant in Surabaya City?"
indexPath: "/path/to/your/vector/index"
Conclusion
The integration of HNSW Vector index with Genkit significantly enhances the capabilities of Generative AI models by providing enriched context and specific knowledge.
This approach not only improves the accuracy of AI responses but also simplifies the process of knowledge integration, making it a powerful tool for various applications.
By following the steps outlined in this article, you can effectively leverage the HNSW Vector index to build more intelligent and context-aware AI systems in a very short time like instantly!
Hope this helps and see you in the next one!
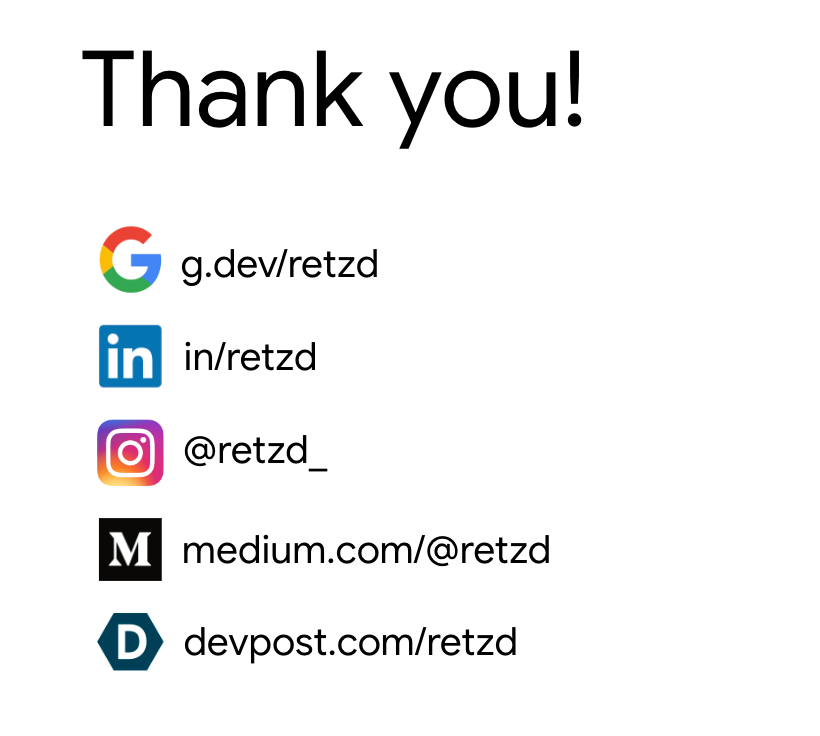
Reliable AI Model Tuning : Leveraging HNSW Vector with Firebase Genkit was originally published in Becoming Human: Artificial Intelligence Magazine on Medium, where people are continuing the conversation by highlighting and responding to this story.
Sentiment Analysis of App Reviews: A Comparison of BERT, spaCy, TextBlob, and NLTK

BERT vs spaCy vs TextBlob vs NLTK in Sentiment Analysis for App Reviews
Sentiment analysis is the process of identifying and extracting opinions or emotions from text. It is a widely used technique in natural language processing (NLP) with applications in a variety of domains, including customer feedback analysis, social media monitoring, and market research.
There are a number of different NLP libraries and tools that can be used for sentiment analysis, including BERT, spaCy, TextBlob, and NLTK. Each of these libraries has its own strengths and weaknesses, and the best choice for a particular task will depend on a number of factors, such as the size and complexity of the dataset, the desired level of accuracy, and the available computational resources.
In this post, we will compare and contrast the four NLP libraries mentioned above in terms of their performance on sentiment analysis for app reviews.
BERT (Bidirectional Encoder Representations from Transformers)
BERT is a pre-trained language model that has been shown to be very effective for a variety of NLP tasks, including sentiment analysis. BERT is a deep learning model that is trained on a massive dataset of text and code. This training allows BERT to learn the contextual relationships between words and phrases, which is essential for accurate sentiment analysis.
BERT has been shown to outperform other NLP libraries on a number of sentiment analysis benchmarks, including the Stanford Sentiment Treebank (SST-5) and the MovieLens 10M dataset. However, BERT is also the most computationally expensive of the four libraries discussed in this post.
spaCy
spaCy is a general-purpose NLP library that provides a wide range of features, including tokenization, lemmatization, part-of-speech tagging, named entity recognition, and sentiment analysis. spaCy is also relatively efficient, making it a good choice for tasks where performance and scalability are important.
spaCy’s sentiment analysis model is based on a machine learning classifier that is trained on a dataset of labeled app reviews. spaCy’s sentiment analysis model has been shown to be very accurate on a variety of app review datasets.
TextBlob
TextBlob is a Python library for NLP that provides a variety of features, including tokenization, lemmatization, part-of-speech tagging, named entity recognition, and sentiment analysis. TextBlob is also relatively easy to use, making it a good choice for beginners and non-experts.
TextBlob’s sentiment analysis model is based on a simple lexicon-based approach. This means that TextBlob uses a dictionary of words and phrases that are associated with positive and negative sentiment to identify the sentiment of a piece of text.
TextBlob’s sentiment analysis model is not as accurate as the models offered by BERT and spaCy, but it is much faster and easier to use.
NLTK (Natural Language Toolkit)
NLTK is a Python library for NLP that provides a wide range of features, including tokenization, lemmatization, part-of-speech tagging, named entity recognition, and sentiment analysis. NLTK is a mature library with a large community of users and contributors.
NLTK’s sentiment analysis model is based on a machine learning classifier that is trained on a dataset of labeled app reviews. NLTK’s sentiment analysis model is not as accurate as the models offered by BERT and spaCy, but it is more efficient and easier to use.
The best NLP library for sentiment analysis of app reviews will depend on a number of factors, such as the size and complexity of the dataset, the desired level of accuracy, and the available computational resources.
BERT is the most accurate of the four libraries discussed in this post, but it is also the most computationally expensive. spaCy is a good choice for tasks where performance and scalability are important. TextBlob is a good choice for beginners and non-experts, while NLTK is a good choice for tasks where efficiency and ease of use are important.
Recommendation
If you are looking for the most accurate sentiment analysis results, then BERT is the best choice. However, if you are working with a large dataset or you need to perform sentiment analysis in real time, then spaCy is a better choice. If you are a beginner or non-expert, then TextBlob is a good choice. If you need a library that is efficient and easy to use, then NLTK is a good choice.
Sentiment Analysis of App Reviews: A Comparison of BERT, spaCy, TextBlob, and NLTK was originally published in Becoming Human: Artificial Intelligence Magazine on Medium, where people are continuing the conversation by highlighting and responding to this story.
CInA: A New Technique for Causal Reasoning in AI Without Needing Labeled Data

Causal reasoning has been described as the next frontier for AI. While today’s machine learning models are proficient at pattern recognition, they struggle with understanding cause-and-effect relationships. This limits their ability to reason about interventions and make reliable predictions. For example, an AI system trained on observational data may learn incorrect associations like “eating ice cream causes sunburns,” simply because people tend to eat more ice cream on hot sunny days. To enable more human-like intelligence, researchers are working on incorporating causal inference capabilities into AI models. Recent work by Microsoft Research Cambridge and Massachusetts Institute of Technology has shown progress in this direction.
About the paper
Recent foundation models have shown promise for human-level intelligence on diverse tasks. But complex reasoning like causal inference remains challenging, needing intricate steps and high precision. Tye researchers take a first step to build causally-aware foundation models for such tasks. Their novel Causal Inference with Attention (CInA) method uses multiple unlabeled datasets for self-supervised causal learning. It then enables zero-shot causal inference on new tasks and data. This works based on their theoretical finding that optimal covariate balancing equals regularized self-attention. This lets CInA extract causal insights through the final layer of a trained transformer model. Experiments show CInA generalizes to new distributions and real datasets. It matches or beats traditional causal inference methods. Overall, CInA is a building block for causally-aware foundation models.
Key takeaways from this research paper:
- The researchers proposed a new method called CInA (Causal Inference with Attention) that can learn to estimate the effects of treatments by looking at multiple datasets without labels.
- They showed mathematically that finding the optimal weights for estimating treatment effects is equivalent to using self-attention, an algorithm commonly used in AI models today. This allows CInA to generalize to new datasets without retraining.
- In experiments, CInA performed as good as or better than traditional methods requiring retraining, while taking much less time to estimate effects on new data.
My takeaway on Causal Foundation Models:
- Being able to generalize to new tasks and datasets without retraining is an important ability for advanced AI systems. CInA demonstrates progress towards building this into models for causality.
- CInA shows that unlabeled data from multiple sources can be used in a self-supervised way to teach models useful skills for causal reasoning, like estimating treatment effects. This idea could be extended to other causal tasks.
- The connection between causal inference and self-attention provides a theoretically grounded way to build AI models that understand cause and effect relationships.
- CInA’s results suggest that models trained this way could serve as a basic building block for developing large-scale AI systems with causal reasoning capabilities, similar to natural language and computer vision systems today.
- There are many opportunities to scale up CInA to more data, and apply it to other causal problems beyond estimating treatment effects. Integrating CInA into existing advanced AI models is a promising future direction.
This work lays the foundation for developing foundation models with human-like intelligence through incorporating self-supervised causal learning and reasoning abilities.
CInA: A New Technique for Causal Reasoning in AI Without Needing Labeled Data was originally published in Becoming Human: Artificial Intelligence Magazine on Medium, where people are continuing the conversation by highlighting and responding to this story.
Machine Learning Revolutionizes Cybersecurity; Detecting, Preventing Threats
Image Embedding, Image Similarity and Caption generation with Live Streamlit implementation
The potential of utilizing unstructured data, particularly image data, in the fashion and lifestyle retail industry is immense. With the…
Continue reading on Becoming Human: Artificial Intelligence Magazine »
Machine Learning Model Identifies Hidden Cases of Hidradenitis Suppurativa
Simplifying AI: A Dive into Lightweight Fine-Tuning Techniques
In natural language processing (NLP), fine-tuning large pre-trained language models like BERT has become the standard for achieving state-of-the-art performance on downstream tasks. However, fine-tuning the entire model can be computationally expensive. The extensive resource requirements pose significant challenges.
In this project, I explore using a parameter-efficient fine-tuning (PEFT) technique called LoRA to fine-tune BERT for a text classification task.
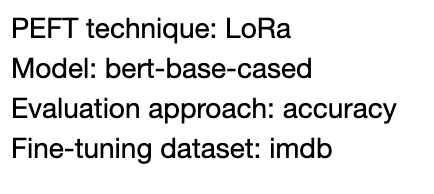
I opted for LoRA PEFT technique.
LoRA (Low-Rank Adaptation) is a technique for efficiently fine-tuning large pre-trained models by inserting small, trainable matrices into their architecture. These low-rank matrices modify the model’s behavior while preserving the original weights, offering significant adaptations with minimal computational resources.
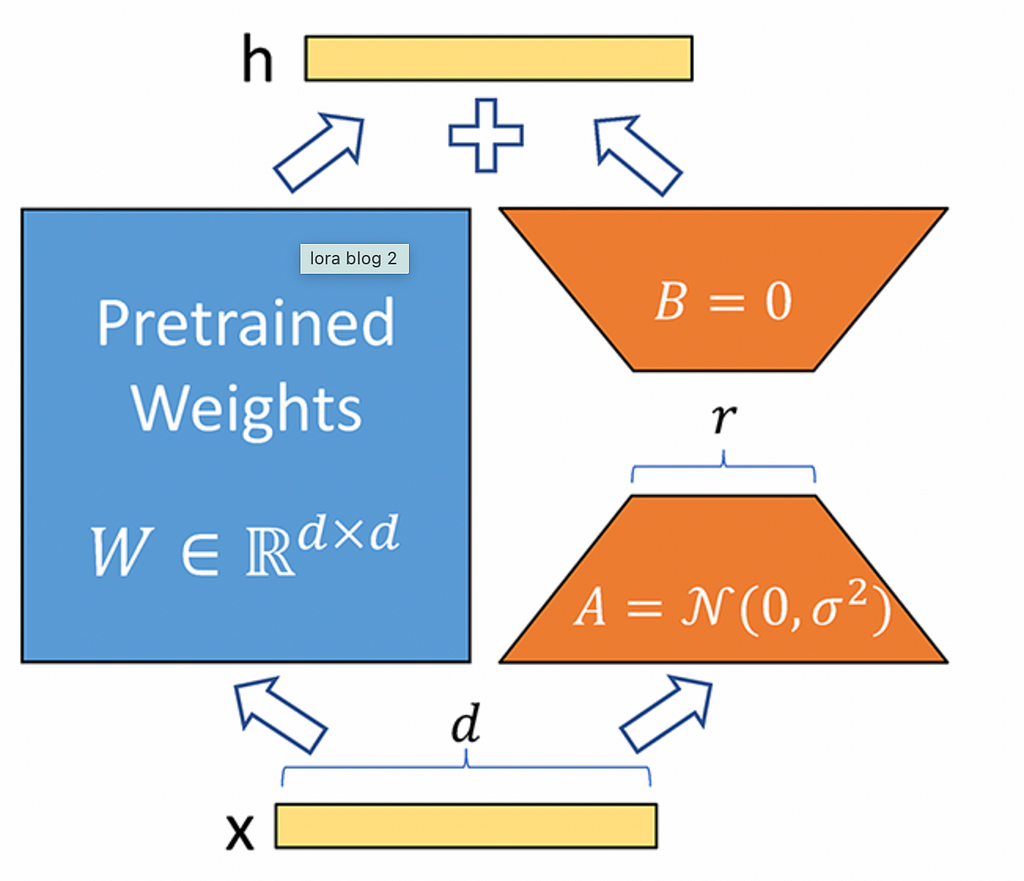
In the LoRA technique, for a fully connected layer with ‘m’ input units and ’n’ output units, the weight matrix is of size ‘m x n’. Normally, the output ‘Y’ of this layer is computed as Y = W X, where ‘W’ is the weight matrix, and ‘X’ is the input. However, in LoRA fine-tuning, the matrix ‘W’ remains unchanged, and two additional matrices, ‘A’ and ‘B’, are introduced to modify the layer’s output without altering ‘W’ directly.
The base model I picked for fine-tuning was BERT-base-cased, a ubiquitous NLP model from Google pre-trained using masked language modeling on a large text corpus. For the dataset, I used the popular IMDB movie reviews text classification benchmark containing 25,000 highly polar movie reviews labeled as positive or negative.
Evaluating the Foundation Model
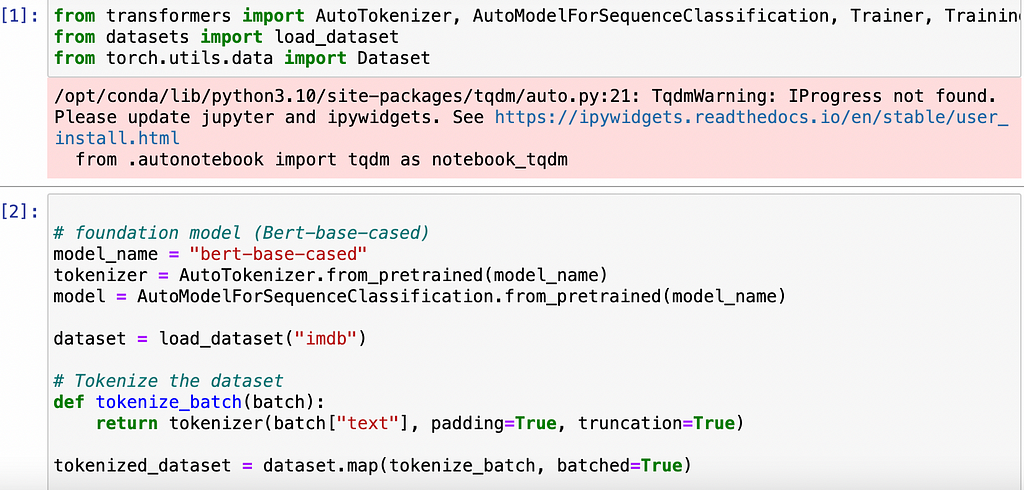

I evaluated the bert-base-cased model on a subset of our dataset to establish a baseline performance.
First, I loaded the model and data using HuggingFace transformers. After tokenizing the text data, I split it into train and validation sets and evaluated the out-of-the-box performance:
The Core of Lightweight Fine-Tuning
The heart of the project lies in the application of parameter-efficient techniques. Unlike traditional methods that adjust all model parameters, lightweight fine-tuning focuses on a subset, reducing the computational burden.

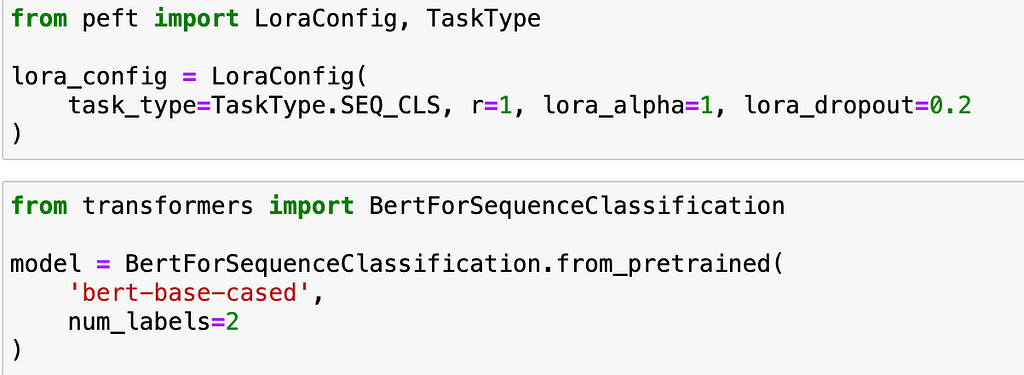
I configured LoRA for sequence classification by defining the hyperparameters r and α. R controls the percentage of weights that are masked, and α controls the scaling applied to the masked weights to keep their magnitude in line with the original value. I masked 80% by setting r=0.2 and used the default α=1.
After applying LoRA masking, I retrained just the small percentage of unfrozen parameters on the sentiment classification task for 30 epochs.

LoRA was able to rapidly fit the training data and achieve 85.3% validation accuracy — an absolute improvement over the original model!
Result Comparision
The impact of lightweight fine-tuning is evident in our results. By comparing the model’s performance before and after applying these techniques, we observed a remarkable balance between efficiency and effectiveness.
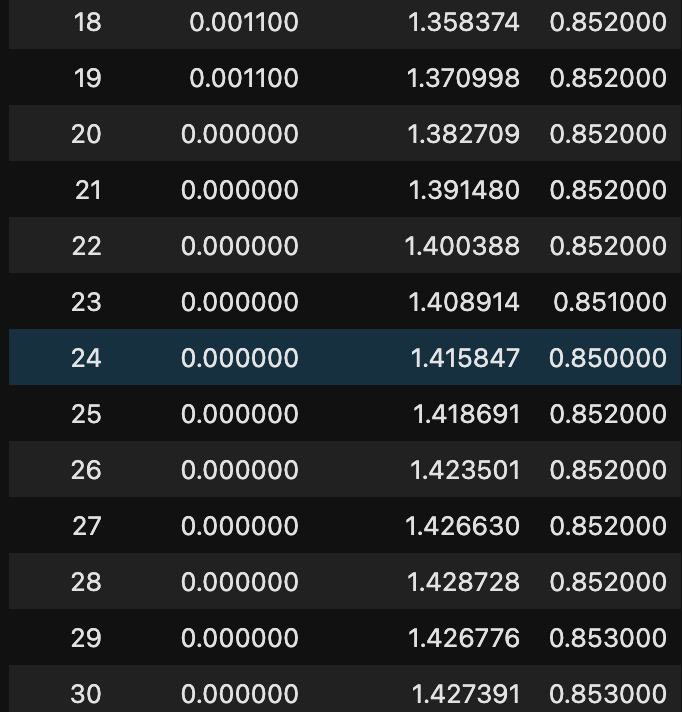
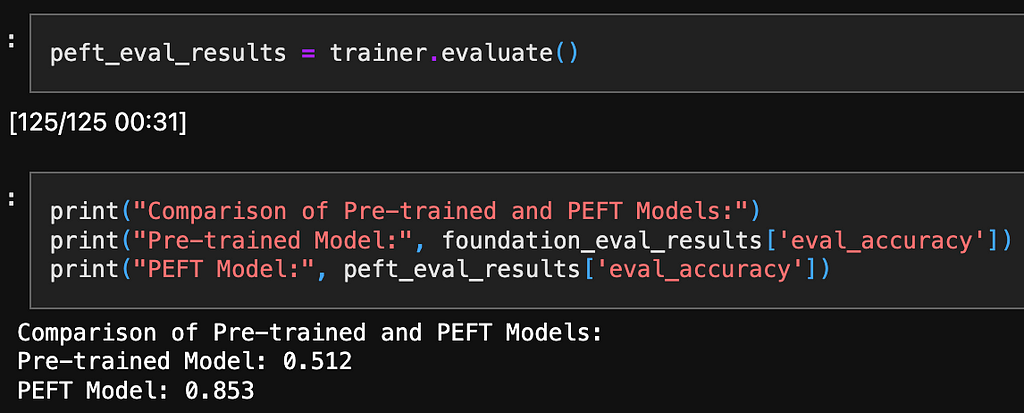
Results
Fine-tuning all parameters would have required orders of magnitude more computation. In this project, I demonstrated LoRA’s ability to efficiently tailor pre-trained language models like BERT to custom text classification datasets. By only updating 20% of weights, LoRA sped up training by 2–3x and improved accuracy over the original BERT Base weights. As model scale continues growing exponentially, parameter-efficient fine-tuning techniques like LoRA will become critical.
Other methods in the documentation: https://github.com/huggingface/peft
Simplifying AI: A Dive into Lightweight Fine-Tuning Techniques was originally published in Becoming Human: Artificial Intelligence Magazine on Medium, where people are continuing the conversation by highlighting and responding to this story.
How to collect voice data for machine learning
Machine learning and artificial intelligence have revolutionized our interactions with technology, mainly through speech recognition systems. At the core of these advancements lies voice data, a crucial component for training algorithms to understand and respond to human speech. The quality of this data significantly impacts the accuracy and efficiency of speech recognition models.
Various industries, including automotive and healthcare, increasingly prioritize deploying responsive and reliable voice-operated systems.
In this article, we’ll talk about the steps of voice data collection for machine learning. We’ll explore effective methods, address challenges, and highlight the essential role of high-quality data in enhancing speech recognition systems.

Understanding the Challenges of Voice Data Collection
Collecting speech data for machine learning faces three key challenges. They impact the development and effectiveness of machine learning models. These challenges include:
Varied Languages and Accents
Gathering voice data across numerous languages and accents is a complex task. Speech recognition systems depend on this diversity to accurately comprehend and respond to different dialects. This diversity requires collecting a broad spectrum of data, posing a logistical and technical challenge.
High Cost
Assembling a comprehensive voice dataset is expensive. It involves costs for recording, storage, and processing. The scale and diversity of data needed for effective machine learning further escalate these expenses.
Lengthy Timelines
Recording and validating high-quality speech data is a time-intensive process. Ensuring its accuracy for effective machine learning models requires extended timelines for data collection.
Data Quality and Reliability
Maintaining the integrity and excellence of voice data is key to developing precise machine-learning models. This challenge involves meticulous data processing and verification.
Technological Limitations
Current technology may limit the quality and scope of voice data collection. Overcoming these limitations is essential for developing advanced speech recognition systems.
Methods of Collecting Voice Data
You have various methods available to collect voice data for machine learning. Each one comes with unique advantages and challenges.
Prepackaged Voice Datasets
These are ready-made datasets available for purchase. They offer a quick solution for basic speech recognition models and are typically of higher quality than public datasets. However, they may not cover specific use cases and require significant pre-processing.
Public Voice Datasets
Often free and accessible, public voice datasets are useful for supporting innovation in speech recognition. However, they generally have lower quality and specificity than prepackaged datasets.
Crowdsourcing Voice Data Collection
This method involves collecting data through a wide network of contributors worldwide. It allows for customization and scalability in datasets. Crowdsourcing is cost-effective but may have equipment quality and background noise control limitations.
Customer Voice Data Collection
Gathering voice data directly from customers using products like smart home devices provides highly relevant and abundant data. This method raises ethical and privacy concerns. Thus, you might have to consider legal restrictions across certain regions.
In-House Voice Data Collection
Suitable for confidential projects, this method offers control over the data collection, including device choice and background noise management. It tends to be costly and less diverse, and the real-time collection can delay project timelines.
You may choose any method based on the project’s scope, privacy needs, and budget constraints.
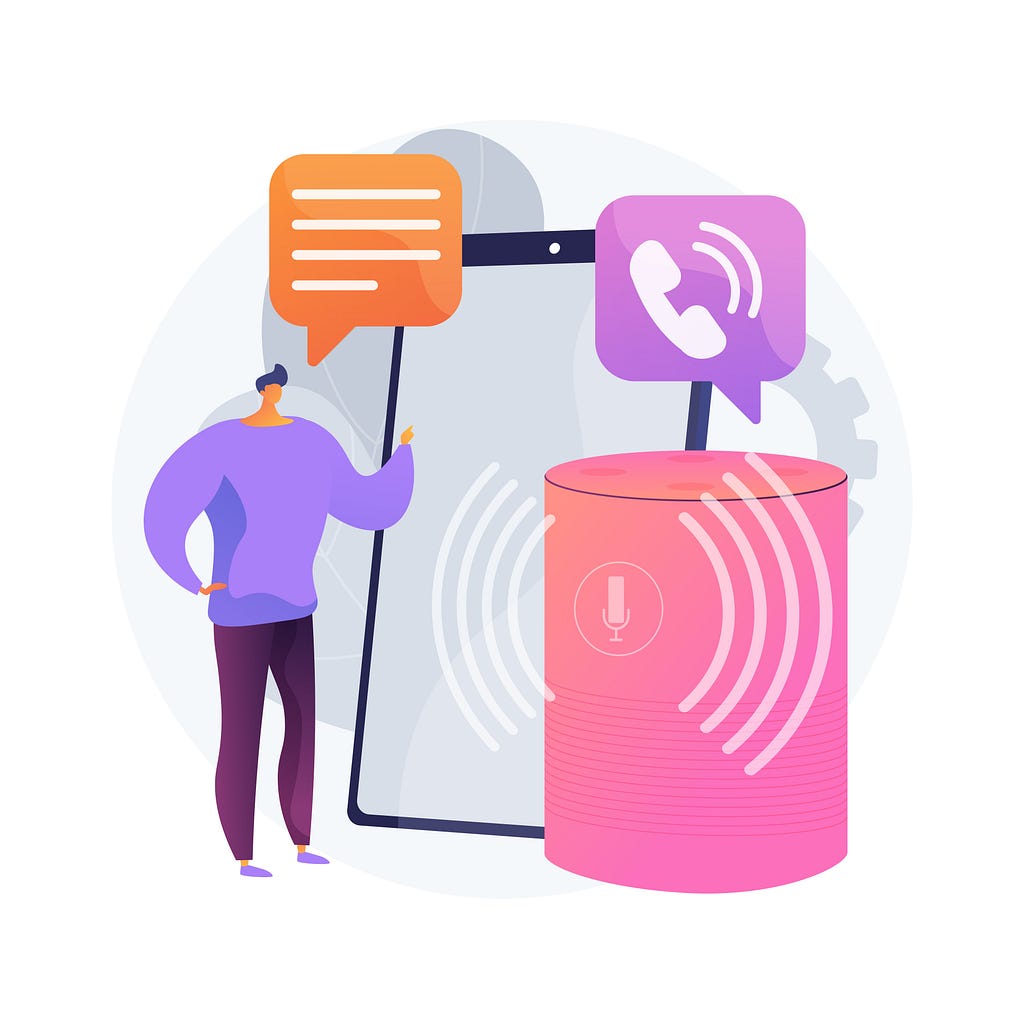
Exploring Innovative Use Cases and Sources for Voice Data
Voice data is essential across various innovative applications.
- Conversational Agents: These agents, used in customer service and sales, rely on voice data to understand and respond to customer queries. Training them involves analyzing numerous voice interactions.
- Call Center Training: Voice data is crucial for training call center staff. It helps with accent correction and improves communication skills which enhance customer interaction quality.
- AI Content Creation: In content creation, voice data enables AI to produce engaging audio content. It includes podcasts and automated video narration.
- Smart Devices: Voice data is essential for smart home devices like virtual assistants and home automation systems. It helps these devices comprehend and execute voice commands accurately.
Each of these use cases demonstrates the diverse applications of voice data in enhancing user experience and operational efficiency.
Bridging Gaps and Ensuring Data Quality
We must actively diversify datasets to bridge gaps in voice data collection methodologies. This includes capturing a wider array of languages and accents. Such diversity ensures speech recognition systems perform effectively worldwide.
Ensuring data quality, especially in crowdsourced collections, is another key area. It demands improved verification methods for clarity and consistency. High-quality datasets are vital for different applications. They enable speech systems to understand varied speech patterns and nuances accurately.
Diverse and rich datasets are not just a technical necessity. They represent a commitment to inclusivity and global applicability in the evolving field of AI.

Ethical and Legal Considerations in Voice Data Collection
Ethical and legal considerations hold a lot of importance when collecting voice data, particularly from customers. These include:
- Privacy Concerns: Voice data is sensitive. Thus, you need to respect the user’s privacy.
- Consent: Obtaining explicit consent from individuals before collecting their voice data is a legal requirement in many jurisdictions.
- Transparency: Inform users about how you will use their data.
- Data Security: Implement robust measures to protect voice data from unauthorized access.
- Compliance with Laws: Adhere to relevant data protection laws, like GDPR, which govern the collection and use of personal data.
- Ethical Usage: Make sure you use the collected data ethically and do not harm individuals or groups.
Conclusion
The field of voice data collection for machine learning constantly evolves, facing new advancements and challenges. Key takeaways from this discussion include:
- Diverse Data Collection: Emphasize collecting varied languages and accents for global applicability.
- Cost-Benefit Analysis: Weigh the costs against the potential benefits of comprehensive datasets.
- Time Management: Plan for extended timelines due to the meticulous nature of data collection and validation.
- Legal and Ethical Compliance: Prioritize adherence to privacy laws and ethical standards.
- Quality Over Quantity: Focus on the quality and reliability of data for effective machine learning.
- Technological Adaptation: Stay updated with technological developments to enhance data collection methods.
These points show the dynamic nature of voice data collection. They highlight the need for innovative, ethical, and efficient approaches to machine learning.
How to collect voice data for machine learning was originally published in Becoming Human: Artificial Intelligence Magazine on Medium, where people are continuing the conversation by highlighting and responding to this story.
Five Machine Learning Types to Know
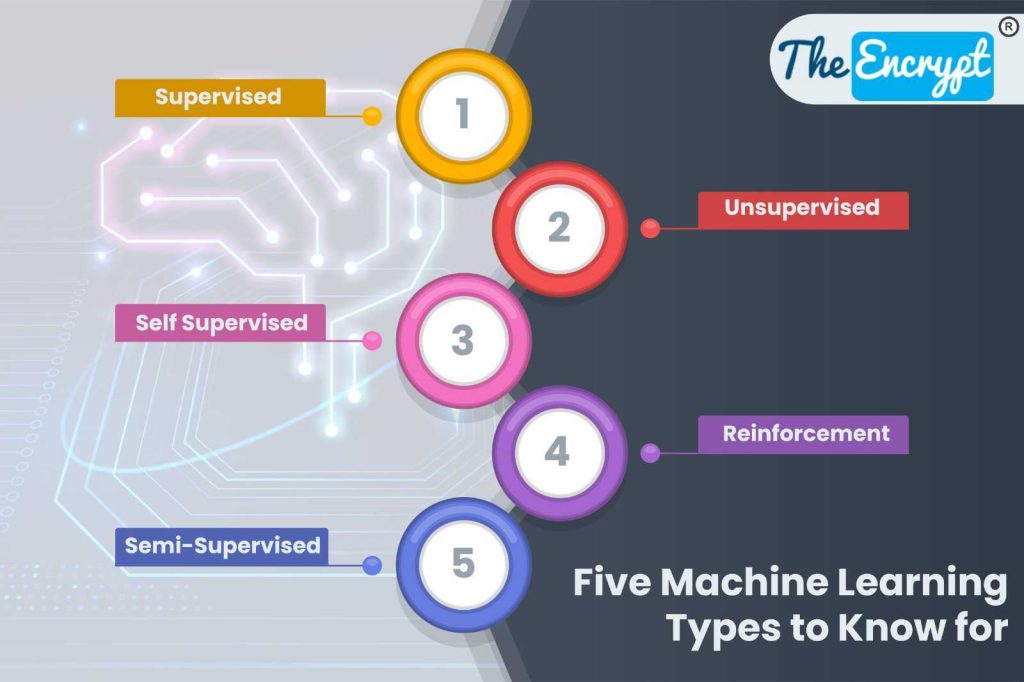

The concept of machine learning is completely changing the world and revolutionizing various sectors. But did you know that there are different types of machine learning as well? The concept behind it is to ensure that systems can learn and improve data without the need for programming. Its algorithms are quite varied, and each caters […]
The post <strong>Five Machine Learning Types to Know</strong> appeared first on The Encrypt – Tech News & Updates.
Why Traditional Machine Learning is relevant in LLM Era?
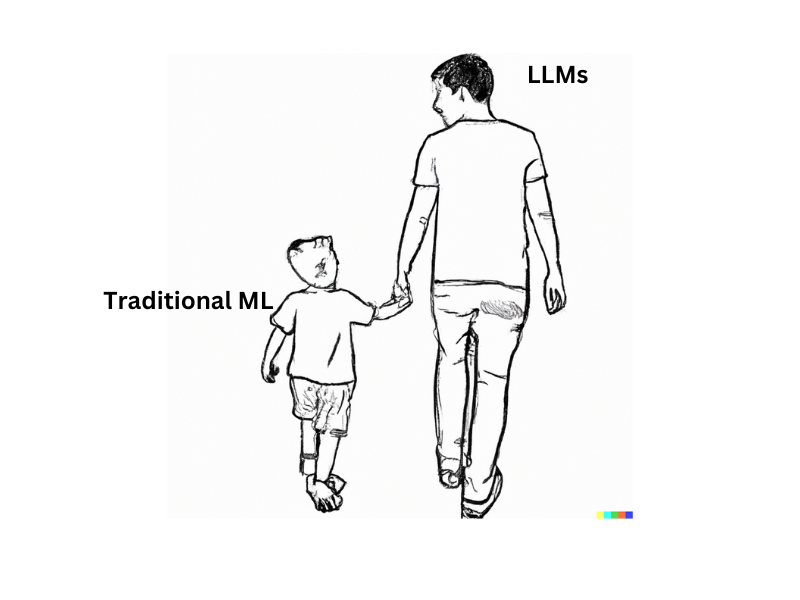
Day to day, we are witnessing a significant adoption of LLMs in academia and industry. You name any use case, and the answer is LLMs. While I’m happy about this, I’m concerned about not considering traditional machine learning and deep learning models like logistic regression, SVM, MLP, LSTMs, autoencoders, etc., depending on the use case. As we do in machine learning by first getting it done with a baseline model and developing on top of it, I would say if the use case has the best solution with a small model, we should not be using LLMs to do it. This article is a sincere attempt to give some ideas on when to choose traditional methods over LLMs or the combination.
“It’s good to choose a clap to kill a mosquito than a sword”
Data:
- LLMs are more hungry for data. It is important to strike a balance between model complexity and the available data. For smaller datasets, we should go ahead and try traditional methods, as they get the job done within this quantity. For example, the classification of sentiment in a low-resource language like Telugu. However, when the use case has less data and is related to the English language, we can utilize LLMs to generate synthetic data for our model creation. This overcomes the old problems of the data not being comprehensive in covering the complex variations.
Interpretability:
- When it comes to real-world use cases, interpreting the results given by models holds considerable importance, especially in domains like healthcare where consequences are significant, and regulations are stringent. In such critical scenarios, traditional methods like decision trees and techniques such as SHAP (SHapley Additive exPlanations) offer a simpler means of interpretation. However, the interpretability of Large Language Models (LLMs) poses a challenge, as they often operate as black boxes, hindering their adoption in domains where transparency is crucial. Ongoing research, including approaches like probing and attention visualization, holds promise, and we may soon reach a better place than we are right now.
Computational Efficiency:
- Traditional machine learning techniques demonstrate superior computational efficiency in both training and inference compared to their Large Language Model (LLM) counterparts. This efficiency translates into faster development cycles and reduced costs, making traditional methods suitable for a wide range of applications.
- Let’s consider an example of classifying the sentiment of a customer care executive message. For the same use case, training a BERT base model and a Feed Forward Neural Network (FFNN) with 12 layers and 100 nodes each (~0.1 million parameters) would yield distinct energy and cost savings.
- The BERT base model, with its 12 layers, 12 attention heads, and 110 million parameters, typically requires substantial energy for training, ranging from 1000 to 10,000 kWh according to available data. With best practices for optimization and a moderate training setup, achieving training within 200–800 kWh is feasible, resulting in energy savings by a factor of 5. In the USA, where each kWh costs $0.165, this translates to around $165 (10000 * 0.165) — $33 (2000 * 0.165) = $132 in cost savings. It’s essential to note that these figures are ballpark estimates with certain assumptions.
- This efficiency extends to inference, where smaller models, such as the FFNN, facilitate faster deployment for real-time use cases.
Specific Tasks:
- There are use cases, such as time series forecasting, characterized by intricate statistical patterns, calculations, and historical performance. In this domain, traditional machine learning techniques have demonstrated superior results compared to sophisticated Transformer-based models. The paper [Are Transformers Effective for Time Series Forecasting?, Zeng et al.] conducted a comprehensive analysis on nine real-life datasets, surprisingly concluding that traditional machine learning techniques consistently outperformed Transformer models in all cases, often by a substantial margin. For those interested in delving deeper. Check out this link https://arxiv.org/pdf/2205.13504.pdf
Hybrid Models:
- There are numerous use cases where combining Large Language Models (LLMs) with traditional machine learning methods proves to be more effective than using either in isolation. Personally, I’ve observed this synergy in the context of semantic search. In this application, the amalgamation of the encoded representation from a model like BERT, coupled with the keyword-based matching algorithm BM25, has surpassed the results achieved by BERT and BM25 individually.
- BM25, being a keyword-based matching algorithm, tends to excel in avoiding false positives. On the other hand, BERT focuses more on semantic matching, offering accuracy but with a higher potential for false positives. To harness the strengths of both approaches, I employed BM25 as a retriever to obtain the top 10 results and used BERT to rank and refine these results. This hybrid approach has proven to provide the best of both worlds, addressing the limitations of each method and enhancing overall performance.
In conclusion, based on your usecase it might be a good idea to experiment traditional machine learning models or hybrid models keeping in consideration of interpretation, available data, energy and cost savings along with the possible benefits of combining them with llms. Have a good day. Happy learning!!
Thanks to all blogs, generative ai friends bard, chatgpt for helping me 🙂
Until next time, cheers!
Why Traditional Machine Learning is relevant in LLM Era? was originally published in Becoming Human: Artificial Intelligence Magazine on Medium, where people are continuing the conversation by highlighting and responding to this story.